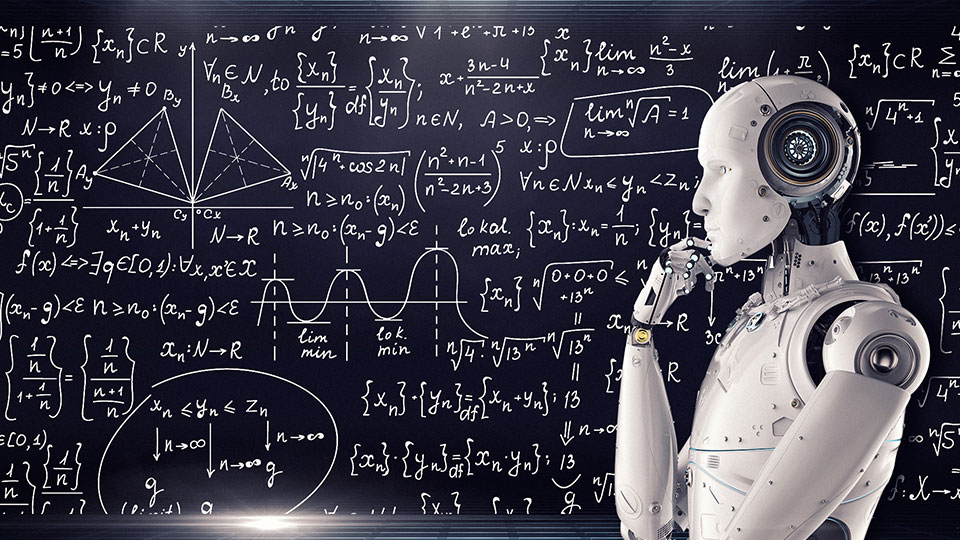
Following a successful launch event in November, the IAS Annual Theme 'AI: Facts, Fictions, Futures' continues with a hybrid event based at the Loughborough London Campus.
Our Fellows share fascinating stories of scientific discovery made possible by Artificial Intelligence, from the design of engineering materials to drug molecules. They explore how AI techniques transcend discipline boundaries and open a new world of discovery.
How can we go beyond the perception of AI as a “dark art”, and build transparent discovery routes that win public trust? How can we communicate effectively both across disciplines and to our wider global community? These questions (and more) receive a worthwhile airing in this event.
Convened by: Jin Xuan, Valerie Pinfield, Amalia Sabiescu
IAS Visiting Fellows in residence:
Ankit Agrawal, (Virtual) Northwestern University
Gareth Conduit, (In-Person) Cambridge University
Raffaella Ocone, (In-Person) Heriot-Watt University
Isao Tanaka, (Virtual) Kyoto University
Programme:
10.00 |
Refreshments and welcome |
10.30 |
Introductory Remarks Prof Steve Rothberg PVCR Prof Jin Xuan |
10.40 |
Data driven discovery of new inorganic materials Prof Isao Tanaka, Kyoto University |
11.30 |
Responsible Technologies and Innovations Prof Raffaella Ocone, Heriot-Watt University |
12.20 |
Lunch and informal discussions |
13.05 |
Reconvene |
13.10 |
The modern-day blacksmith Dr Gareth Conduit, University of Cambridge |
14.00 |
Artificial Intelligence and High-Performance Data Mining Dr Ankit Agrawal, Northwestern University |
14.50 |
Concluding remarks |
15.00 |
Refreshments and informal discussions |
Isao Tanaka, Kyoto University (Online)
Data driven discovery of new inorganic materials
Discovery of inorganic oxides and nitrides with the aid of data centric technique will be presented. One of the approaches uses materials database that is generated by first principles density functional theory (DFT) calculations. The other uses experimental datasets obtained in our robot-based laboratory where a large number of parallel experiments are performed.
Raffaella Ocone, Heriot-Watt University (In person)
Responsible Technologies and Innovation
The development and adoption of emerging technologies and digitalisation demand for ethical considerations and responsible innovation. Ethical assessment of specific products, uses, regulations, and social impacts of entrenched technologies might be simplified by the fact that it can rely on a wide range of available data leading to ethical synthesis and reasoning; on the contrary, the ethics of emerging technologies can only make use of speculative data about future products and processes, their envisaged use and their impact.
The talk explores of the effects that innovation can bring to the environment, the human being and society at large suggesting some synthesis and solutions that developers and users must adopt to assure a responsible employment of emerging technologies.
Gareth Conduit, University of Cambridge (In person)
The modern-day blacksmith
We present a machine learning methodology, Alchemite, that exploits multiple sources of materials information: experimental data, physical laws, and computer simulations. We illustrate the tool by designing a nickel-base superalloy for direct laser deposition. Starting from a training set comprising just eight results, the machine learning tool juxtaposes complementary material properties to circumvent missing data. We design an alloy and the associated processing parameters, and then present the experimental validation of its properties. We highlight further case studies in materials, healthcare, and pharmaceuticals that motivated the commercialization of the machine learning tool by Intellegens.
Ankit Agrawal, Northwestern University (Online)
Artificial Intelligence and High-Performance Data Mining for Accelerating Scientific Discovery
The increasing availability of data from the first three paradigms of science (experiments, theory, and simulations), along with advances in artificial intelligence and machine learning (AI/ML) techniques has offered unprecedented opportunities for data-driven science and discovery, which is the fourth paradigm of science. Within the arena of AI/ML, deep learning (DL) has emerged as a game-changing technique in recent years with its ability to effectively work on raw big data, bypassing the (otherwise crucial) manual feature engineering step traditionally required for building accurate ML models, thus enabling numerous real-world applications, such as autonomous driving. In this talk, I will present our ongoing research in AI and high performance data mining, along with illustrative real-world scientific applications. In particular, we will discuss approaches to gainfully apply DL on big data (by accelerating DL and enabling deeper learning) as well as on small data (deep transfer learning) in the context of materials science. I will also demonstrate some of the software tools developed in our group.
Please Note -
If you wish to attend in-person at the London Campus, please click here.
If you wish to attend virtually, please click here.
(AI image file is licensed under the Creative Commons Attribution 2.0 Generic license - https://creativecommons.org/licenses/by/2.0/deed.en
Author - mikemacmarketing, Image via www.vpnsrus.com)
Contact and booking details
- Name
- Kieran Teasdale
- Email address
- K.Teasdale@lboro.ac.uk
- Cost
- Free
- Booking required?
- Yes