Research and innovation
Our vision is to provide world leading engineering solutions to today’s global challenges.
We are passionate about our research and continually strive to strengthen and stimulate our portfolio. Our industrially focused research enables us to work with some of the world’s most renowned engineering and commercial companies.
The breadth of our research is captured in our research priorities which are served with activities from our research groups and a number of cutting-edge research centres and institutes that are hosted by the School.
Our doctoral graduates are testament to our success, and through our strong links with organisations around the globe, we ensure our students’ skills, knowledge and research remain competitive and relevant to build a better future for all.
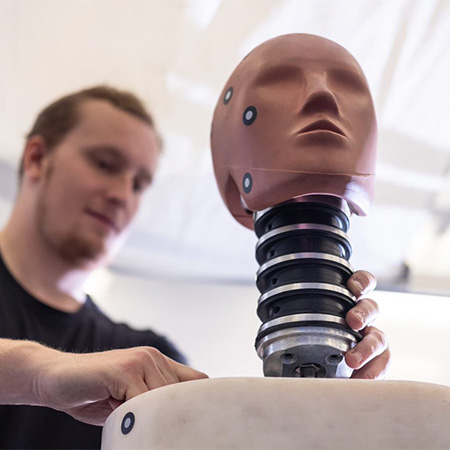
Research spotlights
Shining a light on some of the largest and most impactful research projects taking place within the School of Mechanical, Electrical and Manufacturing Engineering, from changing the ICC’s cricket helmet policy to applying our modelling and simulation research to support the development of new PV standards.